DiffTalk
DiffTalk: Crafting Diffusion Models for Generalized Audio-Driven Portraits Animation
Shuai Shen Wenliang Zhao Zibin Meng Wanhua Li Zheng Zhu Jie Zhou Jiwen Lu
Tsinghua University
[Paper] [Code] [Video]
Video
Abstract & Method
Talking head synthesis is a promising approach for the video production industry. Recently, a lot of effort has been devoted in this research area to improve the generation quality or enhance the model generalization. However, there are few works able to address both issues simultaneously, which is essential for practical applications. To this end, in this paper, we turn attention to the emerging powerful Latent Diffusion Models, and model the Talking head generation as an audio-driven temporally coherent denoising process (DiffTalk). More specifically, instead of employing audio signals as the single driving factor, we investigate the control mechanism of the talking face, and incorporate reference face images and landmarks as conditions for personality-aware generalized synthesis. In this way, the proposed DiffTalk is capable of producing high-quality talking head videos in synchronization with the source audio, and more importantly, it can be naturally generalized across different identities without any further fine-tuning. Additionally, our DiffTalk can be gracefully tailored for higher-resolution synthesis with negligible extra computational cost. Extensive experiments show that the proposed DiffTalk efficiently synthesizes high-fidelity audio-driven talking head videos for generalized novel identities.
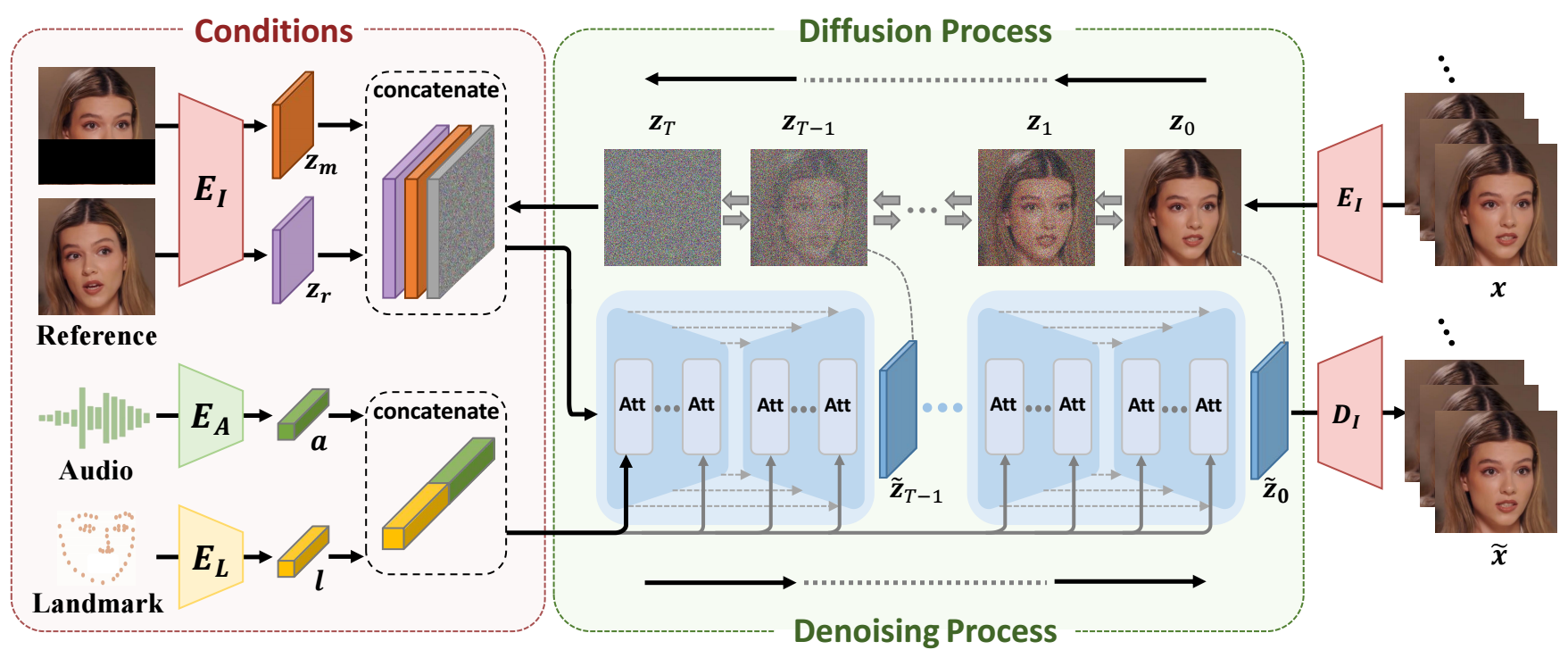
Results
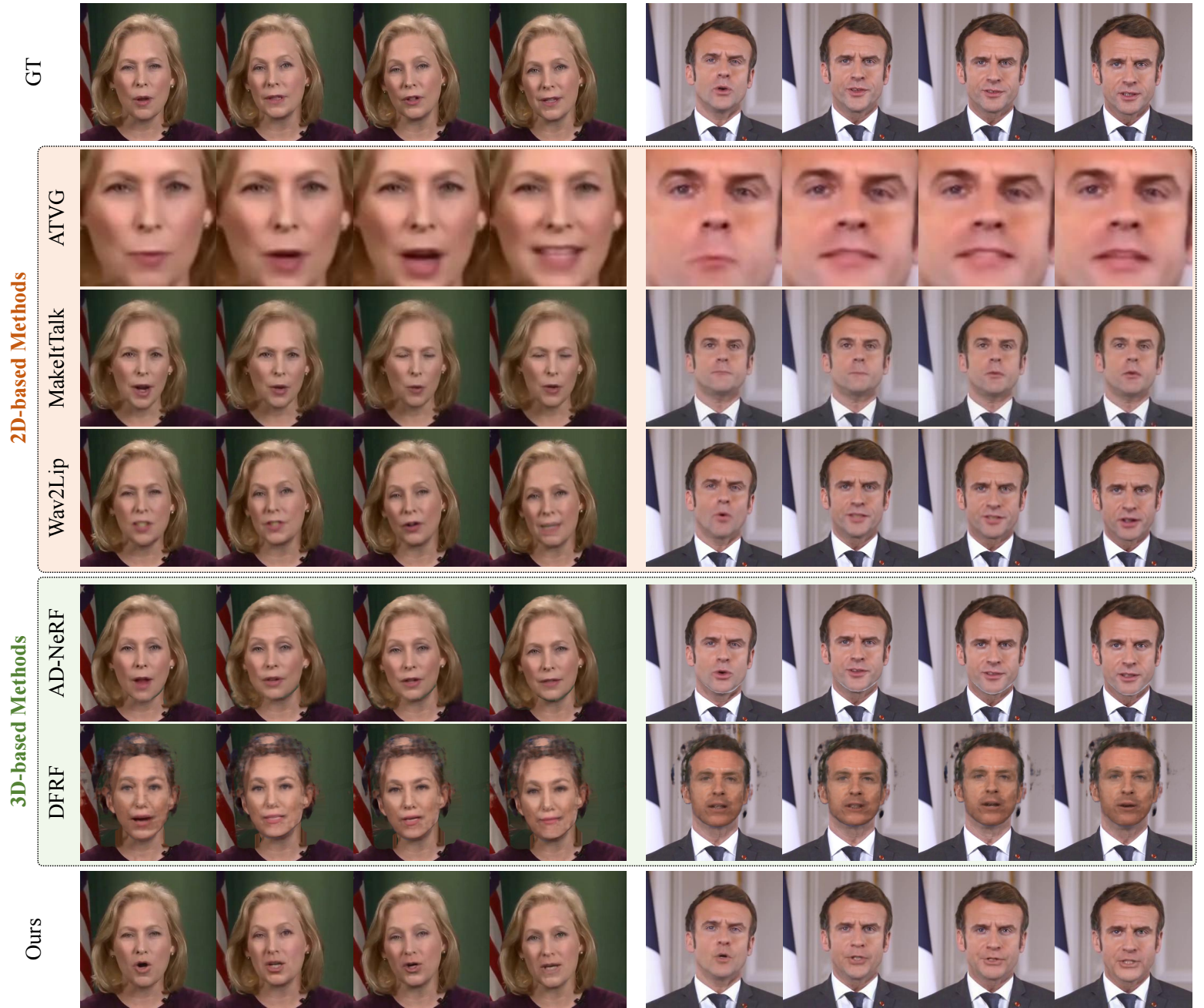
Citation
Shuai Shen, Wenliang Zhao, Zibin Meng, Wanhua Li, Zheng Zhu, Jie Zhou, Jiwen Lu. "DiffTalk: Crafting Diffusion Models for Generalized Audio-Driven Portraits Animation."
In CVPR 2023.
Bibtex
|